AI-driven Automated Structural Health Monitoring (see full blog here)
Mechanics-Informed Autoencoder Enables Automated Detection and Localization of Unforeseen Structural Damage
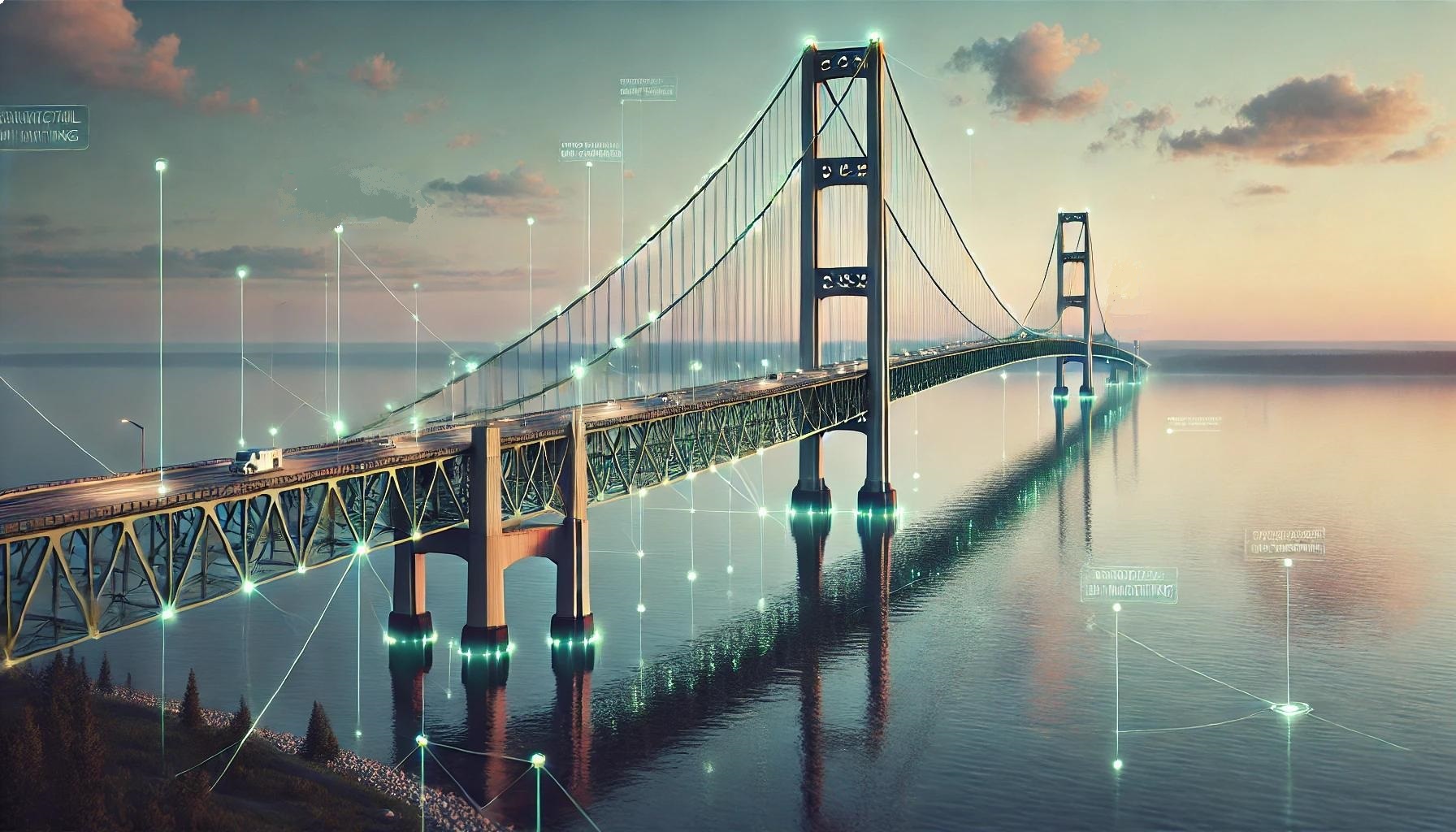
See blog post at Nature Research Communities Infrastructure resilience is emerging as a crucial need in the face of increased risks and hazards impacts. This highlights the need for reliable, cost-effective, and scalable infrastructure condition assessment and monitoring approaches. The need is driven by recent real-world failures that have led to catastrophic consequences, from the unnoticed cracks in the I-40 Bridge in Memphis, which resulted in an emergency closure, to the collapse of a bridge in Pittsburgh in 2022 due to undetected corrosion. These incidents reveal the limitations of traditional inspection methods and emphasize the need for more intelligent and efficient solutions. To prevent future catastrophes, it is essential to develop accurate baseline models that are scalable, generalizable, and tailored to the unique characteristics of each structure. AI-driven automated Structural Health Monitoring (SHM) systems offer a promising avenue to address the shortcomings of existing manual inspections and sensor technologies. By leveraging advanced algorithms and machine learning techniques, these systems can enhance early detection and localization of structural damage, thereby significantly improving infrastructure resilience and safety.